From principles to practice: Responsible AI in action
How can companies unlock the value in artificial intelligence while mitigating the downsides? We asked leaders in this quickly changing field to weigh in.
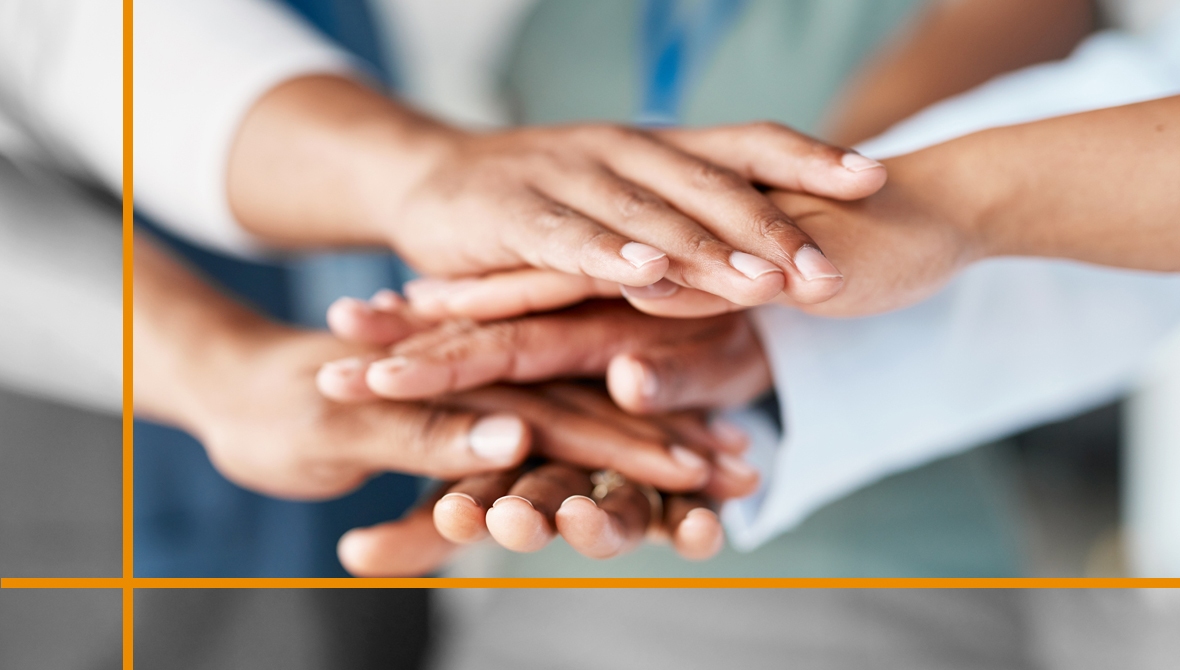
The combination of rapid-fire advancements in artificial intelligence and moves to integrate it into an array of business-critical systems has put a spotlight on using the technology responsibly. PwC’s 27th Annual Global CEO Survey captures the combination of palpable excitement and understandable caution felt by leaders regarding the newest wave of AI innovation—generative AI (GenAI). Almost one-third of respondents said their organizations had already implemented this nascent technology, and nearly 60% expected it to improve their company’s products within the next 12 months. However, they also foresaw an increase in associated risks. Though cybersecurity topped the list of their worries, CEOs also expressed concern about the threat of legal and reputational liabilities and about controlling the spread of misinformation.
Addressing these challenges requires a responsible AI toolkit that helps companies pursue AI’s value while mitigating its downsides (see exhibit: “A responsible AI toolkit provides a foundation for trust-building practices”). However, to truly grasp the essence of these principles, it’s instructive to see how they’re implemented in real-world scenarios. To gain this perspective, we engaged with a diverse group of executives attending the fall 2023 World Summit AI conference in Amsterdam. Each leader we spoke with approaches responsible AI from a unique vantage point, whether it’s as a technology practitioner grappling with practical challenges, a business executive formulating strategy, or an entrepreneur seeking to offer critical AI risk mitigation tools to businesses.
Building the foundation
Responsible AI starts with drafting a core set of principles that are grounded in, or at least clearly aligned with, company values. Khagesh Batra, head of data science at HR services company the Adecco Group, draws on the ethical principles set forth by his organization to guide his work with AI. “Corporations offer trainings when you are onboarded,” said Batra. “Most people tend to glance through them, but they actually contain those guiding principles. What are your company values? What is discrimination? They don’t explicitly state [them] for data scientists, but I feel like you can automatically infer it.”
Marc Mathieu, head of AI transformation at Salesforce, agreed that embedding responsible AI in organizational values lays the foundation. “It very much is part of our values. Our culture is driven all the way from the top and throughout the organization. Trusted AI is really one of the core elements that Salesforce has been focusing on.”
Driving accountability
Mathieu went on to explain that companies can’t simply stop there. “Principles are great, but it’s in the implementation that things go wrong,” Mathieu said.
“We’ve got an ethics team that has created guidelines to make sure we give a North Star to customers, partners, and [employees] internally on how to implement AI strategies and deployments in a way that’s responsible and ethical. But at the same time, there is the product team that’s developing the actual technical solutions to make sure that we can guarantee trust in generative AI. We ensure these teams are very much in sync [with each other] as well as with our research team. We often say that trust is not just a layer; it’s a road map. Build that muscle of trusted, ethical AI now, because the road map is going to, if anything, become more and more complex as we go toward autonomous AI.”
Trust is not just a layer; it’s a road map. Build that muscle of trusted, ethical AI now, because the road map is going to become more and more complex as we go toward autonomous AI.”
Accountability extends beyond ethics and product teams to the technologists at the forefront of AI development, such as those sourcing the data used to train generative AI and other AI models. As Batra told us, “Responsible AI is paramount, because at the end of the day, you could be dealing with sensitive data that could have a lot of impact.”
Putting principles to work for privacy, safety, and bias mitigation
In practice, one of the challenges for data practitioners is to collect data that represents a diverse population and doesn’t introduce—or perpetuate—bias. Sarah Porter, a UN advisor on autonomous weapons and human rights, and founder of Inspired Minds, a global 200,000-member emerging tech community, articulated the particular challenge posed by large language models underpinning generative systems. “One of the problems that we have with large language models is that we are training them with massive historical datasets,” she said. “You can say we are re-creating the very worst of history. We need to ensure that the information we’re feeding into these machines represents the outcomes that we want in terms of diversity, which is difficult to do.”
At times, this requires data science professionals to make trade-offs. “We want to use variables that have an impact in the model, but don’t discriminate,” said Batra. “So sometimes I have to make a conscious choice to let go of some performance and say, ‘I’m not going to deal with variables like race or gender in my model because they perpetuate the differentiation that the data has seen, and that would continue if I built them in the model.’”
There are ways to root out bias that also tackle other challenges such as ensuring data privacy. One of these approaches is red-teaming, which in the context of AI refers to a process in which a group deliberately attempts to challenge, attack, or exploit an AI system to identify its vulnerabilities or potential for misuse. Said Mathieu, “We’re using some very interesting methods like red-teaming, not just for privacy, but also for toxicity, inclusion, and bias to make sure that we really go out of the way in delivering trust.”
Solutions that enable technologists to extract the value from sensitive data while anonymizing personal information offer another option for strengthening data privacy. One example of this sort of solution came from Patricia Thaine, who founded Private AI, a company that detects sensitive data and strips it of personal identifiers before it gets shared with an AI model. “Natural language contains some of the most sensitive information you can produce. You can, for example, determine if someone has a medical condition based on how they talk,” she explained. “The great thing about unstructured data is that a lot of the value is around the personal information. For example, to pull out the most important information in a conversation or the sentiment toward a product, you don’t necessarily need to know the speaker.”
It’s also important to avoid training a model on data that contains flat-out harmful material, such as violent imagery. But this is easier said than done. Generative AI models require training on datasets that contain millions of data points. Sorting through it all to ensure the safety of every iota is no easy task. Stability AI’s head of solutions engineering, Tom Mason, told us that his company uses training datasets that are heavily filtered to remove explicit and illegal content. The company is also increasingly turning to AI itself to help confirm that generated content is safe. “At the image generation point, we built an image classifier that uses a computer vision model to classify the image,” said Mason. “If someone manages to generate an image of nudity, for example, the model detects the nudity and blurs it out.”
Designing for trust
Every responsible AI toolkit should emphasize trust by design, which means embedding best practices throughout AI development and deployment processes. This involves going well beyond vetting the data pulsing through a model. And it’s an area in which most companies lag woefully behind, despite the presence of mounting regulations.
“There’s so much to do, even for GDPR compliance that hasn’t been done yet,” said Thaine, referring to the European Union’s General Data Protection Regulation aimed at information privacy. “You can think of the GDPR as an aspirational regulation, where we definitely didn’t have the technology to comply with it when it came out. We still don’t, but we are working toward that,” she said.
“Currently the process [for companies] is kind of manual—that’s the problem,” CTO and cofounder of LatticeFlow, Dr. Pavol Bielik told us. “When we talk to companies, people typically nod and say, ‘Yes, this is the problem I have across my AI stack, and the tools are essentially lagging behind.’ We’ve been working on this for ten years, and from a technological standpoint, these are non-trivial things.” LatticeFlow is one of several players aiming to fill the technological void with a platform that can identify the root causes of model and data issues in an automated fashion throughout the build and deployment process.
“With safety and trust, you have to do it from the beginning,” Dr. Bielik went on to explain. “In the current state, when we work with many banks, for example, they want to do audits. It’s a postmortem analysis. The question is: how can you move it to the beginning, where the minute you start working with AI, you are already following the best practices, you are already following the guidelines, and at every step, you are getting red flags that say ‘No, this is not how you should be doing it’?”
Dr. Bielik concedes that it’s a significant effort—but it’s one worth making. “It’s just a huge investment. When we talk to more mature companies, they know they need this because they learned the hard way. Where more education is needed is with early-stage AI adopters who have not seen the resulting problems yet, and view this as additional investment. But when you get to a certain scale, you just cannot get by without this. In the long term it makes you faster, even if in the short term you spend more time making sure things work.”
The same can be said for responsible AI more broadly. Companies feel pressure to move quickly to take advantage of the efficiency and innovation AI offers, but doing so without proper guardrails in place can ultimately slow them down—or worse yet, cause significant societal harm. Embedding responsible AI in the company mindset and practitioner toolkit can pave a path for both safety and success.