Data and decisions in soccer
The beautiful game is in a race for off-the-field talent that can deliver a competitive edge through big data.
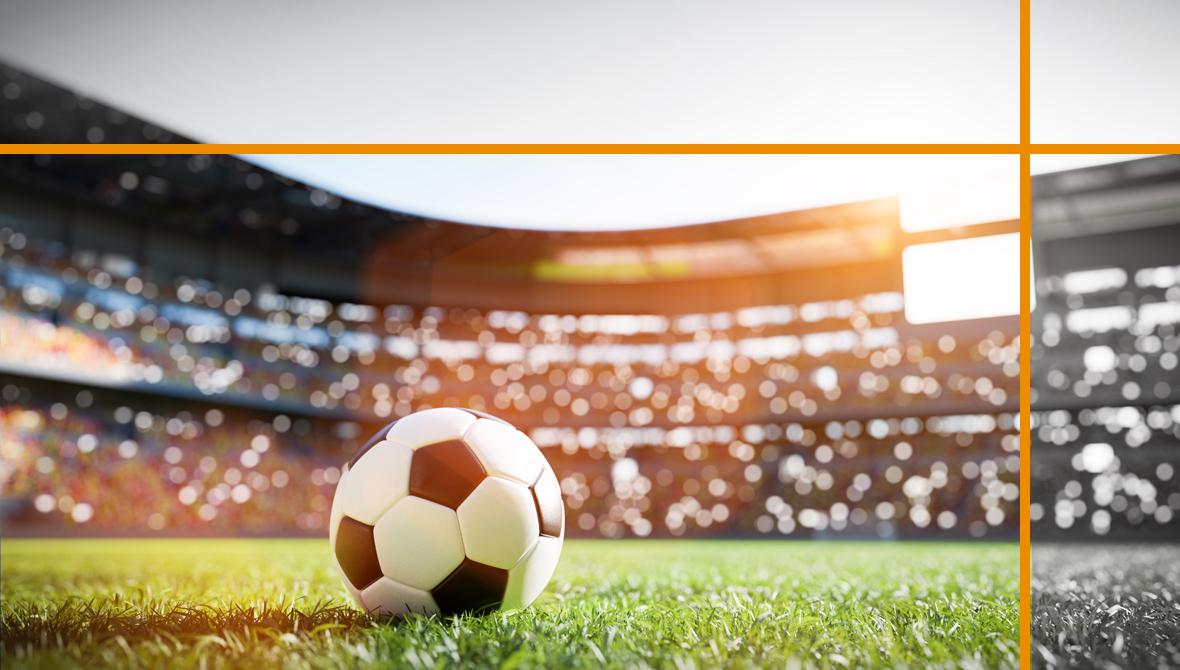
As the 2022 FIFA World Cup gets underway in Qatar on November 20, some of the most important action will be taking place off the field. Most teams will be furiously crunching data on goalies’ tendencies to try to determine how to win a penalty shoot-out if there’s a draw at the game’s final whistle. But this type of single-instance analysis is only a small part of the revolution taking place in the boardrooms at some of soccer’s biggest clubs. Today, the most important hire is no longer the 30-goal-a-season striker or an imposing brick wall of a defender. Instead, there’s an arms race for the person who identifies that talent.
The research department at Liverpool FC, the team that won England’s Premier League in 2020, for example, is now led by a Cambridge University–trained polymer physicist. Arsenal FC recently hired a former Facebook software engineer as a data scientist, and current Premier League champion Manchester City hired a leading AI scientist with a PhD in computational astrophysics to their research department. Chelsea FC’s new American owner, Todd Boehly, spent his summer trying and failing to hire a new sporting director with a data background. These are all examples from England, where the sport’s richest clubs are investing to gain an edge—and often recruiting from ahead-of-the-curve clubs with proven track records, like Monaco, in the French League, and the German club RB Leipzig.
Soccer has a rich history of this sort of analysis. Charles Reep, a military accountant, became soccer’s first data analyst in the 1950s, predating personal computers, Billy Beane, and the Moneyball moment in baseball, in 2003. That was followed up, in 2009, by the soccer equivalent, Soccernomics, by Simon Kuper and Stefan Szymanski, and data-driven sports analysis entered a new era. Among Kuper and Szymanski’s findings: goalkeepers are undervalued in the transfer market, and players from Brazil are overvalued.
I cofounded a football consultancy ten years ago with the authors of the book. One of our first clients was the Netherlands national team. We’ve been applying data to soccer for a while—but a lot of it is backward-looking, trying to mine past performance to account for what could happen on the field. We provided the Dutch team with a penalty-kick dossier before the 2010 World Cup final against Spain, in which Professor Ignacio Palacios-Huerta, an expert in game theory, showed penalty trends and patterns of Spain’s kickers. Spain scored four minutes before the end of the game to win, but the Dutch were confident they would have won on penalties.
Ahead of this World Cup, all teams will be well-versed in what to do in the case of a shoot-out.
Forward pass
The next step in soccer analytics is to use data to develop and project the development of the players themselves, a much more complicated prospect. New data analyses are trying to answer questions about the future by measuring players’ distance run, sprints made, speeds clocked, and their position on the field and expected goals, assists, and threats.
Understanding how data can predict future performance is the challenge facing all decision-makers—those who pay vast sums in transfer fees for players and those who select the teams for games. For example: if a striker scores 25 goals in Holland’s top division, how many would they score in England’s Premier League, where the quality of play is higher (another fact proven by data)? If you’ve paid over the odds for a player who doesn’t perform, it can affect the bottom line. And data helps on a daily basis too: a winger has played six games in three weeks and has muscle soreness—can we predict future injuries, and when should they be rested? What is the “goal probability added” value of a certain pass? Where can value be found in the transfer market?
Understanding how data can predict future performance is the challenge facing all decision-makers—those who hire players and those who select the teams for games.
The traditional ways of measuring a coach’s performance can now be improved, too. An expected goals score line—which predicts a score based on data analysis—can show the nature of a match more clearly than the actual score, where luck or a bad refereeing decision can decide a result. So a coach under pressure, like Leeds United’s American coach Jesse Marsch, can claim, using data analysis, that expected goals is a better indicator of the team’s underlying performance.
Before the covid pandemic, I attended soccer data conferences where mathematics graduates in their early 20s gave presentations on the importance of player orientation in build-up play, or the effect of a particular league on player output. Fast-forward two years, and many of these speakers work in data departments at professional clubs—and are now more reluctant to share their ideas.
One Premier League club that doesn’t want to be named uses what it calls a valuation calculator. This tool works out each player’s market value (MV), which accounts for natural biases like position, nationality, current team, and age, and compares it to their intrinsic value (IV), which evaluates their actual value-add on the field. In an industry in which teams can outperform rivals by buying and selling players smartly in the transfer market—something that you don’t see in other sports, like American football, for example—this tool can help both a selling club (by identifying players with high MV and low IV) and a buying club (by finding players with high IV and low MV).
Some clubs are more willing to talk about their use of data. FC Midtjylland has won the Danish league three times since 2014. The team says it’s down to the numbers: by signing undervalued players like midfielder Tim Sparv and hiring a set-piece coach and a throw-in coach, they gained a competitive advantage. Premier League clubs Brentford FC and Brighton & Hove Albion FC, both owned by men with backgrounds in the betting industry, use data to inform their decisions, as does the Red Bull conglomerate of clubs, to great success.
A few clubs in Europe have also started measuring intangible attributes that are harder to see, like resilience and composure. There are data apps to improve biomechanics, cognitive processing, and tactical performance. Chelsea, for example, measured the psychology of player performance by charting confidence, focus, and motivation-based actions through data.
On the field, this approach is transforming how the game is played: developing various, and better, routines for set pieces, such as corner kicks and spot kicks, provides teams with a clear opportunity to score more goals, as Tottenham Hotspur have done this season (a division-leading ten goals from corners in 15 games) since hiring set-piece specialist Gianni Vio. We also see this new thinking reflected in the rarity of a long-distance screamer shot scoring from outside the penalty area; as the numbers show, the optimal shooting location is nearer the goal, so the average shot distance has dropped. Not surprisingly, players have been told to shoot only when they are nearer the goal. Data is also changing how coaches are selected, as clubs hire tactics-specific coaches to fit their squad’s playing profile.
Soccer is, of course, an emotional sport—it relies on instinct, passion, and character. So it’s natural that there would be a backlash to the data revolution. But for the best outcomes, there is room for both. As Ed Smith, ex-cricketer for England and former head selector for the national cricket team, writes in Making Decisions: Putting the Human Back in the Machine, “Rather than using data instead of human intelligence, the challenge is using data in tandem with the human dimension.” The goal for those using evidence-based decision-making is to use the data to help them understand the game better. Or, as soccer writer Ryan O’Hanlon puts it in his new book, Net Gains: Inside the Beautiful Game’s Analytics Revolution: “Once you think you’ve figured out the answer, someone else will find a better way to ask the question.”
Whether this new approach keeps the beautiful game beautiful is another question. Using data in football, and indeed life, won’t change opinions and shouldn’t preclude human judgment; the point is to find the right data to make the best decisions. And in football, as in life, the game is still searching for the right numbers.